- i24NEWS
- Israel
- Technology & Science
- Israeli study demonstrates high AI accuracy for Crohn's treatment
Israeli study demonstrates high AI accuracy for Crohn's treatment
Professor at Israel's Sheba hospital says 'predicting disease progression and outcomes' is a critical challenge, as he remarks on impact of machine learning
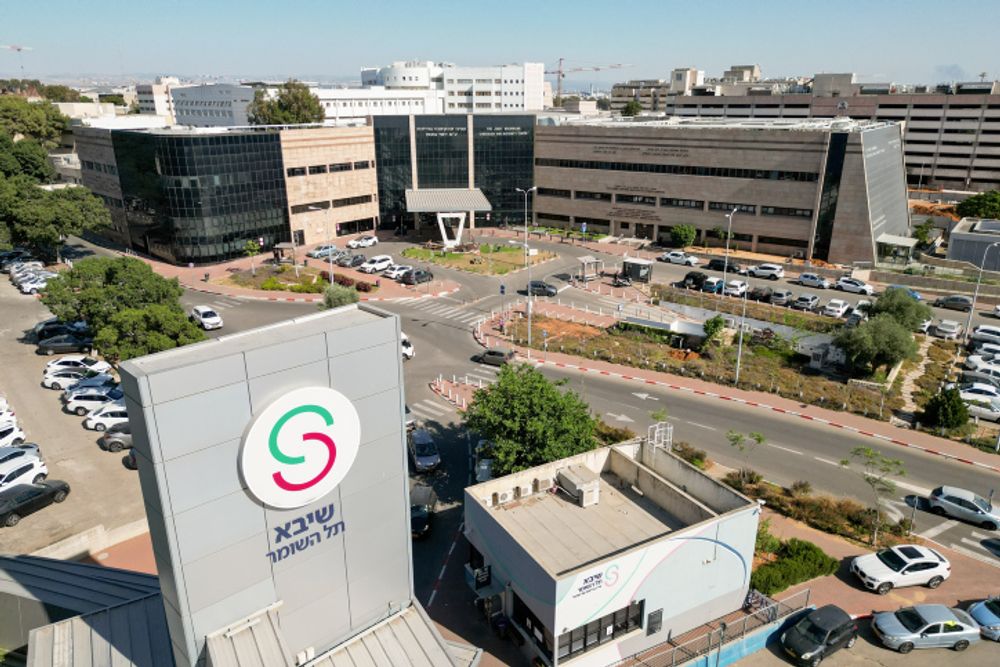
The Sheba Medical Center in Israel announced the publication of peer-reviewed research confirming the accuracy of a newly developed artificial intelligence (AI) algorithm for predicting treatment for Crohn's disease (CD).
"Predicting disease progression and outcomes for patients with Crohn's disease is one of the most critical clinical challenges in the treatment of inflammatory bowel disease (IBD). However, this research highlights the potential impact of AI on this process," said Professor Uri Kopylov, Director of IBD in Sheba's Department of Gastroenterology.
Capsular endoscopy (CE) enables the entire digestive system to be analyzed using a microscopic device equipped with a transmitter and a camera. However, predictors of CD prognosis and response to treatment are still lacking.
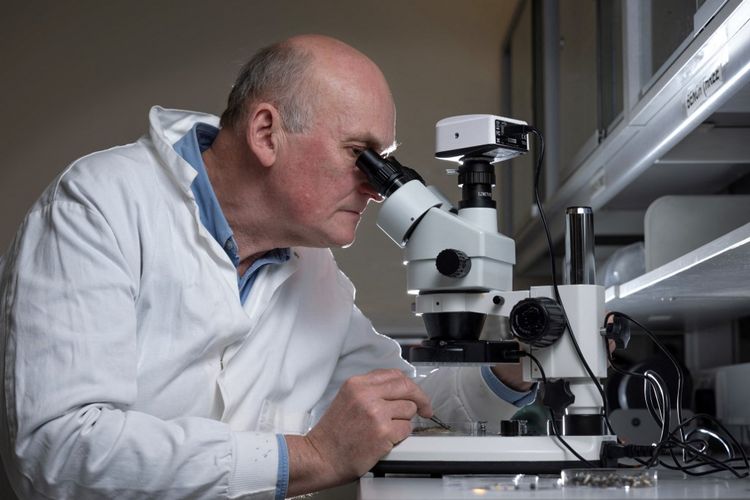
The team from Sheba developed a deep learning model based on CE videos from 101 CD patients, to see if AI could predict the need for biological therapy. The project was led by Professor Kopylov, Professor Shomron Ben-Horin, Department Head of Gastroenterology, and Intel’s Engineering Director Amit Bleiweiss.
The study was published in the journal Therapeutic Advances in Gastroenterology, demonstrating machine-learning analysis as able to accurately predict the need for biological therapy from CE videos at initial diagnosis.
The results of the trial showed that the algorithm was able to predict the need for biological therapy for Crohn's disease patients, with an accuracy of 81-percent. This was significantly higher than a human gastroenterologist analyzing the inflammatory index in stool samples.
This research follows a trial last year, in which AI demonstrated that it could scan a film containing up to 12,000 images in around 2 minutes. In addition, the algorithm was shown to be a highly effective diagnostic tool, producing 86-percent accuracy in image and data analysis, compared with 68-percent accuracy by an experienced gastroenterologist.